Statistical and predictive methods are defined on top of the knowledge graph. Predictive problems include; Impact of drug-drug interactions in survival time, classification of patients. These methods are enhanced with contextual knowledge represented in the knowledge graph with the aim of identifying accurate predictions whose meaning can be described. InterpretME is a tool for fine-grained representations, in a knowledge graph, of the main characteristics of trained machine learning models. InterpretME traces metadata at dfferent stages of a predictive modeling pipeline.
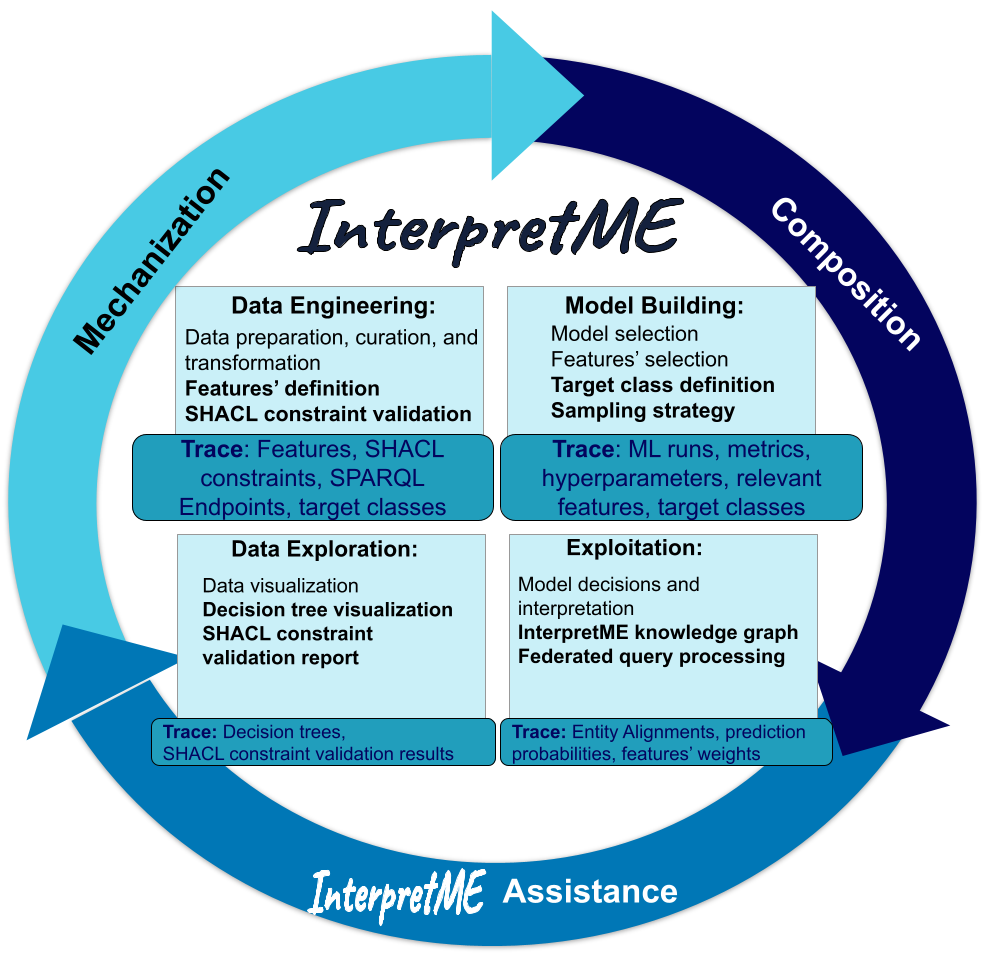
The traced metadata includes features' definition and SHACL validation, and model-based characteristics (e.g., relevant features, and interpretations of prediction probabilities and model decisions). InterpretME allows for the definition of a model's features over knowledge graphs; SHACL states domain integrity constraints. Moreover, InterpretME traces the steps of data collection, curation, integration, and prediction, and documents the collected metadata in a knowledge graph.